The Role of Artificial Intelligence in Advancing Materials Science: Trends and Future Outlook
Materials science is at the heart of numerous technological advancements, from semiconductors and renewable energy to aerospace and biomedical applications. Traditional methods for discovering and optimizing materials rely on experimental trial-and-error, which is time-consuming and resource-intensive. Artificial intelligence (AI) has emerged as a game-changer in materials science, accelerating the discovery of novel materials, optimizing synthesis processes, and predicting material properties with high accuracy. This article explores AI’s transformative role in materials science, supported by recent research and technical data.
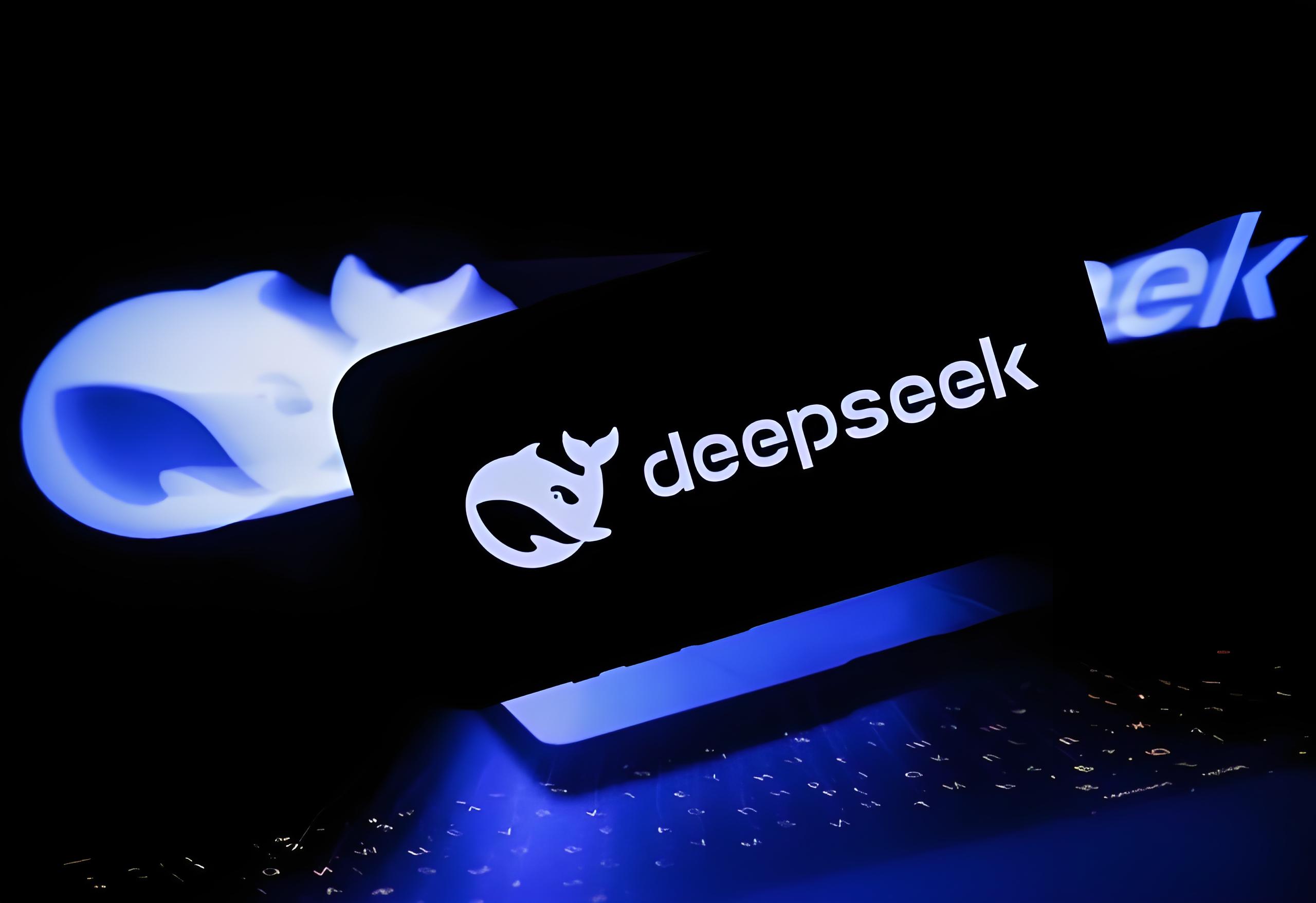
AI in Materials Discovery
One of AI’s most promising applications is in the rapid discovery of new materials. Machine learning (ML) algorithms analyze vast datasets to identify correlations between material structures and properties, significantly reducing the time required for new material development.
AI for Property Prediction and Optimization
AI enhances computational methods like density functional theory (DFT) and molecular dynamics simulations by improving efficiency and accuracy.
AI in Materials Manufacturing and Process Optimization
Beyond material discovery, AI is optimizing manufacturing processes, ensuring high-quality production with minimal waste.
Future Outlook and Challenges
The future of AI in materials science is promising, but challenges remain:
AI is revolutionizing materials science by accelerating discovery, optimizing manufacturing, and enabling unprecedented insights into material properties. As AI models become more advanced and datasets grow, the synergy between AI and materials research will lead to groundbreaking innovations in various industries.
References